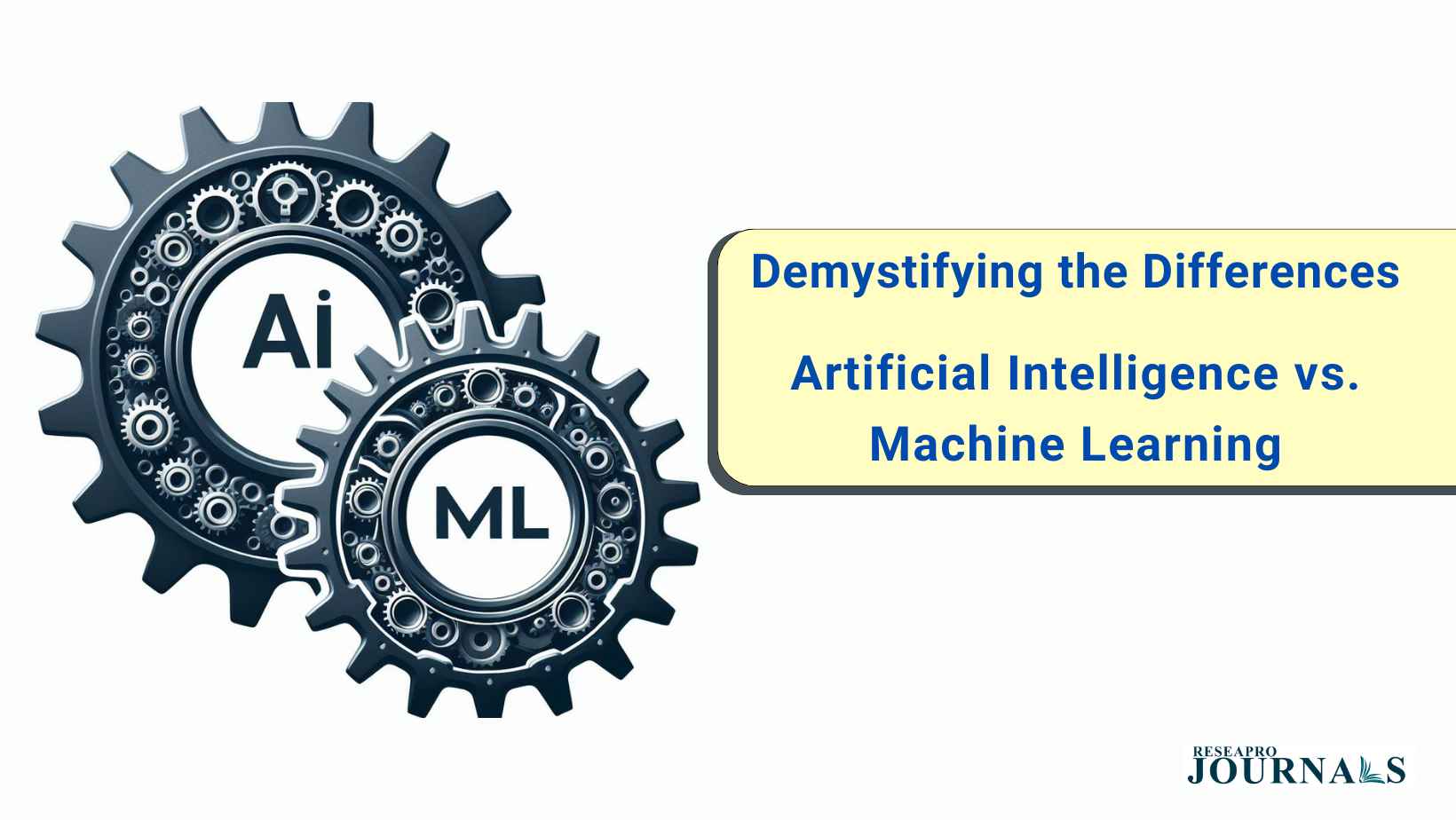
Introduction
Artificial intelligence (AI) and
machine learning (ML) are often used interchangeably, leading to confusion about their distinctions. Understanding these terms is crucial in navigating the rapidly evolving landscape of technology. So, let’s delve into the essence of AI and ML, unraveling their disparities and exploring real-world examples.
What is Artificial Intelligence?
Artificial intelligence refers to the simulation of human intelligence by machines, enabling them to perform tasks that typically require human cognition, such as problem-solving, decision-making, and natural language processing. AI encompasses a broad spectrum of technologies, ranging from rule-based expert systems to
deep learning neural networks.
At its core, AI aims to replicate human-like intelligence in machines, enabling them to perceive their environment, reason about it, and act autonomously to achieve specific goals.
What is Machine Learning?
Machine learning is a subset of artificial intelligence focused on developing algorithms that enable computers to learn from data and improve their performance over time without being explicitly programmed. Unlike traditional rule-based systems, which rely on predefined rules and instructions, machine learning algorithms leverage data to infer patterns, make predictions, and adapt to changing circumstances.
The essence of machine learning lies in its ability to extract meaningful insights from data, discover underlying patterns, and make informed decisions autonomously.
Distinguishing between AI and ML
1. Approach to Problem-Solving:
• AI: Artificial intelligence encompasses various approaches to problem-solving, including rule-based systems, expert systems, and symbolic reasoning. AI systems rely on predefined rules and knowledge bases to make decisions and perform tasks.
• ML: Machine learning, on the other hand, focuses on developing algorithms that can learn from data and improve their performance iteratively. ML algorithms analyze data, identify patterns, and make predictions without explicit programming.
2. Dependency on Data:
• AI: Traditional AI systems rely on predefined rules and instructions encoded by human experts. These systems do not require large amounts of data for operation.
• ML: Machine learning algorithms require substantial amounts of data for training and learning. By analyzing data, ML algorithms can generalize patterns and make predictions on new, unseen data.
3. Autonomy and Adaptability:
• AI: While AI systems can exhibit autonomy in decision-making and task execution, they typically lack adaptability and may struggle to cope with novel situations.
• ML: Machine learning algorithms possess the ability to adapt and learn from new data, making them highly flexible and adaptable to changing environments and circumstances.
Examples of AI and ML in Action
To better understand the distinction between AI and ML, let’s explore some real-world examples:
1. Virtual Personal Assistants:
• AI: Virtual personal assistants like Siri, Alexa, and Google Assistant employ artificial intelligence to understand and respond to user queries, perform tasks, and provide personalized recommendations.
• ML: These assistants utilize machine learning algorithms to improve their speech recognition, natural language understanding, and recommendation capabilities over time. By analyzing user interactions and feedback, they adapt to individual preferences and refine their responses.
2. Image Recognition Systems:
• AI: Image recognition systems utilize artificial intelligence to analyze and interpret visual data, identifying objects, faces, and patterns within images.
• ML: Machine learning algorithms power these systems by learning from labeled images during training. By recognizing patterns and features within images, ML algorithms can classify objects and detect anomalies with high accuracy.
3. Predictive Analytics:
• AI: Predictive analytics systems leverage artificial intelligence to forecast future trends, behaviors, and outcomes based on historical data and statistical models.
• ML: Machine learning plays a central role in predictive analytics by developing models that can identify patterns and correlations within data, enabling accurate predictions and decision-making. For example, ML algorithms can predict customer churn, stock prices, or disease progression based on relevant data inputs.
Conclusion
In summary, while artificial intelligence represents a broad field encompassing various approaches to replicating human-like intelligence in machines,
machine learning represents a specific subset of AI focused on developing algorithms that can learn from data and improve their performance over time. By understanding the distinctions between AI and ML and exploring real-world examples, we can appreciate the transformative potential of these technologies in shaping our future. As we continue to advance in AI and ML research, the possibilities for innovation and progress are limitless, paving the way for a smarter, more connected world.